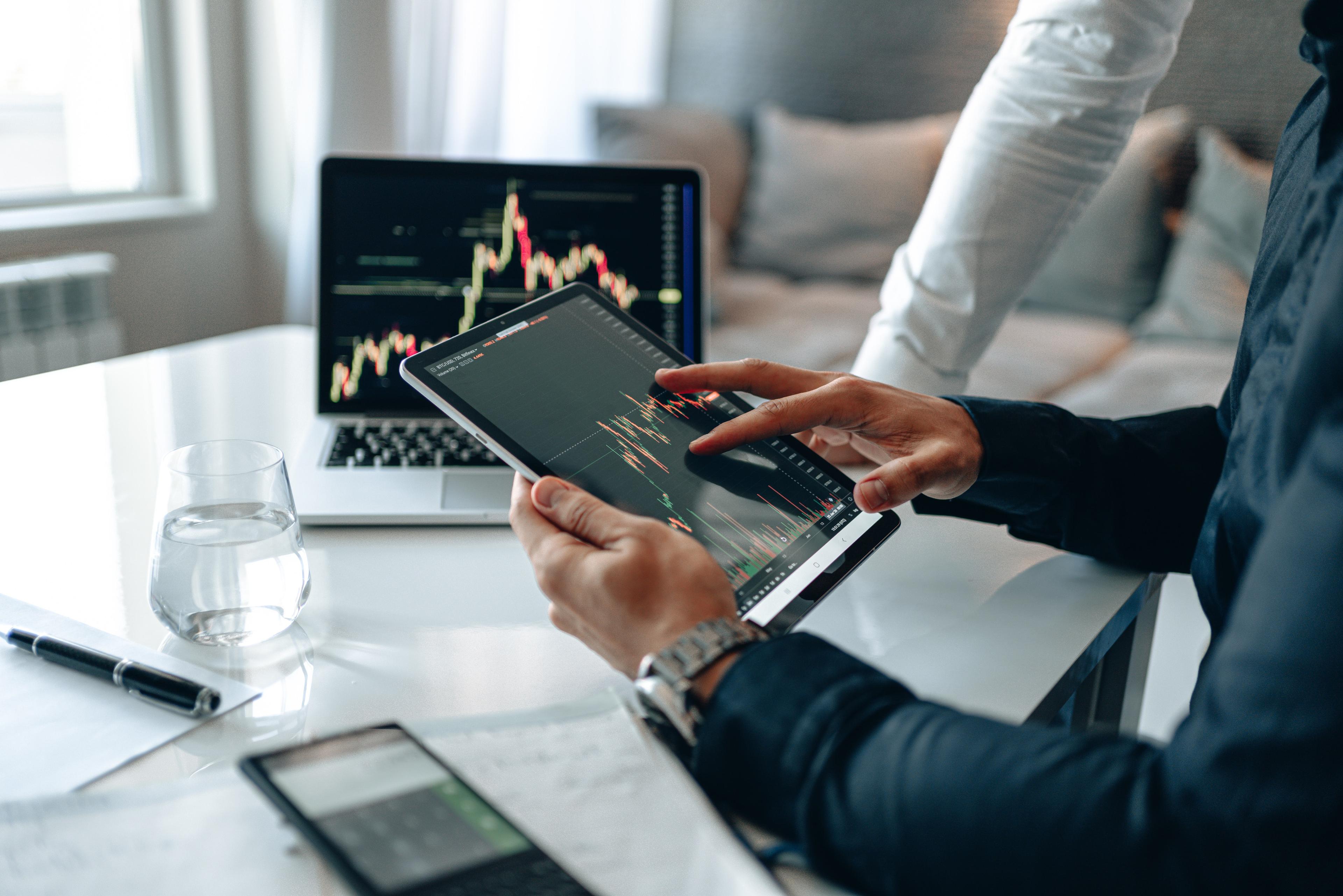
Despite considerable efforts, many companies still struggle to make progress in terms of data management. Based on what we know, there is sufficient awareness of the necessity to use data efficiently, but many companies fail to utilize the available potential. This blog post lists the five most important tips for a successful data management strategy and demonstrates how to implement such a strategy in practice.
Where do you get your data from? Where do you store it? What data types do you differentiate, and how do you analyze these types? By answering these questions, you make the first step towards obtaining an overview of your existing data management.
Challenges associated with data management
However, various challenges make it difficult for companies to answer these questions comprehensively and clearly. The underlying problem: The speed with which data changes, the volume of generated data, and the complexity inherent to the data. In addition, compliance requirements and security aspects make efficient data management even more difficult.
Five current challenges associated with data management are particularly prevalent:
The fragmentation of the data landscape
Ensuring fast access to data while complying with data governance
Legacy architectures, i.e., legacy systems in the data architecture
Advanced analytics increase complexity
Technical tools develop faster than corporate structures
Five tips for a successful data management strategy
You can overcome these challenges by addressing several things in your data strategy.
1. Fragmentation of the data landscape
The problem: If you try to find out where exactly you collect, store, and process your data, you will probably realize that these activities are not contained in a single location. In many companies, you have data lakes, IoT architectures, NoSQL, and graph databases, but also relational databases, which all exist in parallel to each other.
The reason: Various technologies are used, various objectives are pursued, and the scope of collected data varies accordingly.
Tip: Interconnected platforms and technological solutions, such as orchestration layers and interfaces that connect your systems to each other, become a must-have to avoid data fragmentation and make data available across systems.
2. Fast access to data vs. data governance
The problem: Fast access to data is essential if you want to use your data in an expedient manner. On the one hand, data may change within a matter of seconds to indicate new developments. On the other hand, access to data must not be hampered by time-consuming obstacles so that you can optimally integrate it into your day-to-day work. However, if your company has established structured data governance, i.e., if it observes clearly defined rules and processes to maintain data quality and data security, you often encounter problems when it comes to fast and easy access to data.
The reason: The protection of personal and sensitive data should always be at the top of your priorities. This leads to the problem that data is often not accessible to everyone. At the same time, predefined data maintenance or compliance processes specify how you are allowed and required to process data. This can be very complex and time-consuming.
Tip: A key component of modern data governance is the ability to enable fast access to data while ensuring that all relevant data quality, data security, data maintenance, data protection, and corporate compliance requirements are observed. Data access here should be focused on achieving a specific objective. Do you want to adapt your product range to be more in line with customer behavior? If the answer is yes, your aim should be to enable fast access to customer data. For example, you can establish different permissions for different roles to ensure that not everyone can fully view or modify the relevant data.
3. Legacy architectures and legacy systems
The problem: There are usually high expectations when it comes to the analysis and use of data. Justifiably so, since these can offer incredible added value, however, many companies are completely unable to execute the required analyses or fully utilize their data because of old data architectures and legacy systems.
The reason: Unnecessarily complex, isolated data structures often fail to deliver the desired performance with regard to data quality, data processing, access capabilities, and analytics/the further use of data, which is at least in part attributable to the fact that the data structures are not properly interlinked with each other. As a result, companies often work with cobbled-together systems that combine to create a patchwork landscape and are supposed to cover all use cases. However, the output falls far short of the required performance, and the solution simply cannot keep up with the constantly growing requirements.
Tip: In this case, a redesign of the data architecture is unavoidable. This will not only enable you to achieve better results, gain more insights, and utilize the benefits of your data, but more importantly, it will also relieve your coworkers since they will no longer have to spend too much time on maintaining a fractured data architecture and can now focus on more important tasks.
4. Advanced analytics increase complexity
The problem: One of the challenges faced by B2B and B2C companies in all sectors today is that it is no longer sufficient to perform simple data analyses based on past events; rather, you now have to use real-time data or even make forecasts about future developments. The problem is that the existing datasets are often inadequate and cannot furnish the required information. However, new technologies such as machine learning, automation, AI-supported processes, and statistical methods make it possible to generate forecasts. Yet not all companies have the capability to use such advanced analytics.
The reason: The technologies used in advanced analytics are part of what is known as data science. This includes analytical methods for predicting future developments, such as trends or downturns. The aim is to determine the probability of the occurrence of different scenarios and use this information for risk analysis, for example. On the one hand, however, this requires hiring specialist employees, which is often a monumental challenge considering the shortage of skilled workers, and on the other hand, companies need the necessary capacities to implement advanced analytics.
Tip: Evaluate which use cases at your company fall under the scope of advanced analytics. Clarify the importance of these use cases within the framework of your corporate strategy and draw your conclusions if and to what extent you need to invest in advanced analytics to remain competitive and achieve your corporate objectives.
5. Technical tools develop faster than corporate structures
The problem: New technologies appear on the market at an unprecedented rate. Current social crises seem to drive this transformation forward, with the focus in recent years being increasingly placed on data as a currency in a complex environment. Companies are called upon to withstand the growing demands on their data management in order to satisfy customer interests and to be able to meet market challenges in an agile manner. The problem with doing so: Corporate structures are often unable to adapt to technological innovations at the same speed.
The reason: Augmentation, automation, cloud adoption, or data mesh – the new technological trends and changed flows in the technology landscape can only be addressed successfully through appropriate measures at the corporate level. This requires that the corporate structures develop at a similar pace. The areas of business development and business technologies are moving ever closer to each other.
Tip: Companies need a comprehensive view of the critical technological infrastructure. D&A (Data and Analytics) leaders and management personnel must work together to take the necessary measures, implement them on a large scale, as well as measure and evaluate the impact of the managed activities and technologies.
Conclusion: A data management strategy is indispensable
In the same way that your corporate strategy defines how you wish to achieve your economic objectives, a data management strategy defines how you collect and process data in order to make it as useful as possible.
Under the “data management” or “data administration” heading, this strategy encompasses all processes, techniques, and tools with which you manage your company’s data.
This includes data governance, architecture, storage, and backup, but also analytics and data quality. The aim of data management is to collect data in an accessible and secure form. If this is accomplished successfully, your business can enjoy numerous benefits.
Discover more
Lena Füller
Lena drives international editorial and strategic marketing topics including social media, customer references, whitepapers, eBooks and the blog, where she writes about technological trends and digital transformation.