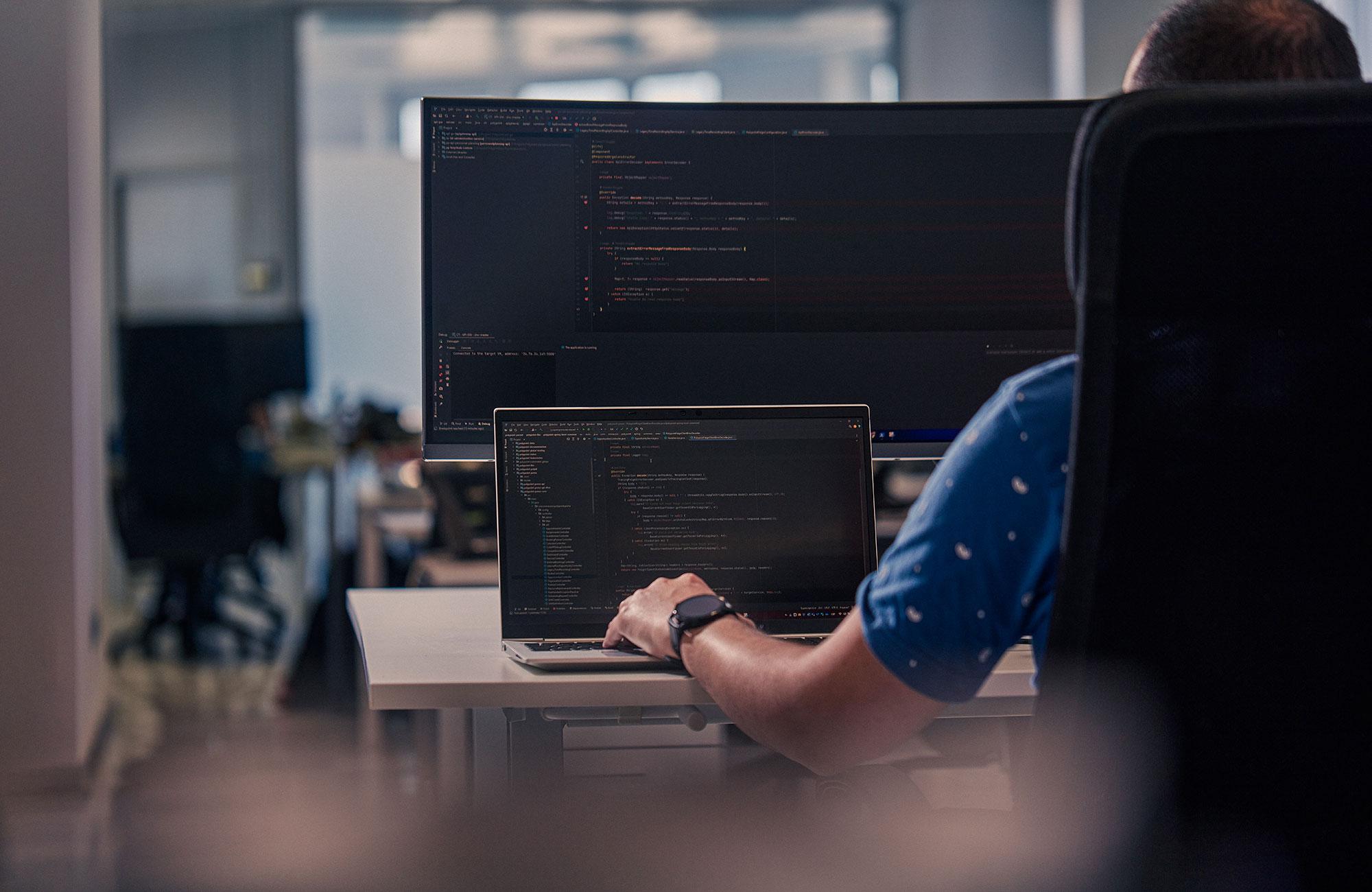
Today, companies see, on the one hand, a growing need for data streaming, but on the other hand, they have to follow new privacy regulations. This dilemma challenges businesses to find intelligent data management techniques that allow them to meet both ends. Therefore, many industries are under pressure to keep the utility of data high while ensuring data security and privacy at all times. The promising techniques they are looking for are called data privacy concepts. This blog post will focus on these concepts and explain how to combine data privacy with the approach of data democratization.
From siloed data to data democratization
Data democratization is a process or a set of initiatives aiming to ease access to data while preserving proper governance of it. The goal is to empower employees to find and use data of interest. This intention shows a significant development and bears much potential for companies. For example, they can improve customer service and support because their service staff can easier access customer data. Also, the performance of processes, machines, or staff can be evaluated and optimized thanks to better data insights that allow companies to get the best out of their operations.
Once an organization is ready to democratize data, shorten business responsiveness and move from single secured storage to real-time streaming, privacy-preserving data pipelines are a must. If a company uses federated data streaming architectures (e.g., in hybrid- or multi-cloud deployments) or even just has a multitude of different data sources, a solid and scalable data streaming solution becomes the fundament for a well-functioning and effective data management.
The relevance of a dedicated data streaming strategy combining data privacy and data democratization
Whether it is finance, healthcare, manufacturing, or any other industry, companies should never thoughtlessly share sensitive data. Unfortunately, this fear of data sharing makes companies turn away from powerful analytics tools in a lot of cases, e.g., offered by Public Cloud (PC) providers or from organizations’ portfolios. However, it doesn’t have to be that way – on the contrary! The challenge is to set up a dedicated data streaming strategy. This strategy must focus on data streaming architectures for efficient and democratized data flow but also create the right environment and structures for safe and secure data transfer.
Data privacy concepts for safe AND democratized data usage
There are plenty of techniques that could help businesses to keep their data safe while at the same time boosting data utilization. Let´s have a look at them in detail:
Define data that needs protection: For companies, it is decisive to filter out and exclude data that shall remain protected from further processing. This is primarily the case for sensitive customer data or corporate numbers and files. In this case, the sensitive data remain siloed and are not part of data streaming flows.
Anonymize and disconnect data: So-called anonymization and perturbation methods allow companies to decouple sensitive data as further analytics rely on de-identified data. Popular solutions here leverage differential privacy algorithms. This is the only way to maintain full control over privacy while using Public Cloud (PC) analytics.
Bring Your Own Key (BYOK) or Hold Your Own Key (HYOK) depending on how stringent the privacy needs are: Encrypting data before sending it to the Cloud and decrypting them after they are back on-premises is one way to ensure secure access to data. This approach facilitates the transition to Public Cloud, however, in the case of HYOK only for storage purposes. HYOK encryption effectively prohibits the usage of PC analytics.
Implementing E2E encryption on the message (or field) level: This concept offers the most fine-grained privacy capabilities. Depending on where the keys are managed, they can prevent PC analytics or can be combined with it. At the same time, this procedure allows for the possibility to stream sensitive data and is GDPR’s right-to-be-forgotten compliant.
Use edge computing as a particular case of pre-processing and filtering of data: Instead of moving all data to the Cloud for PC analytics (i.e., with the help of BYOK), this approach allows the simplified analytics to be moved closer to where the data is generated. Edge computing may be applied in manufacturing as well as in medical telemetry systems (patients’ sensors) in order to apply data masking or implement a simple analysis.
As can be seen, there are plenty of concepts to choose from, and the actual approach can, apart from privacy regulations, refer to many other aspects of an organization’s data strategy. No standard fits all, but companies can, together with an expert like Mimacom, define their individual best practice.
The potential of safe data streaming
Nevertheless, data-stream systems offer much more than only integration and real-time movement of data and go far beyond basic gluing functionality. With real-time machine learning analytics included, stream processing and stream analytics reshape the way the raw data is transformed and used. Stream processing capabilities of data platforms reduce delays and pave the way for real-time actionable insights. Therefore, it is essential to realize its full potential and apply privacy-preserving solutions to data “in use.”
This is how you can democratize data in a secure infrastructure
Robust and scalable privacy-preserving stream systems are not straightforward to design, and finding the right balance between utility and privacy is also challenging. Fortunately, the area is being actively researched, and more and more ideas and tools are appearing. Nevertheless, at the beginning of the democratization program, it is good to follow a few basic rules:
Start small: Choose a single business domain, maybe even only a part of it. Choose a subset of privacy regulations the data needs to comply with. Decide what kind of end-to-end privacy approach is the most suitable one. Develop PoC solutions and, make sure they remain expandable, challenge them within an organization’s community.
Let data privacy be part of a data governance program: Data privacy is very important but only a part of a much more comprehensive data governance program. Define goals and a vision for your data platform to utilize the best methods for privacy and management.
Engage with a broader community: Moving away from centralized silos to federated data platforms could potentially lead to independent, disconnected islands of partial solutions. To avoid that and retain data potential, data governance shall be conceptualized together with all interested parties. Hence, building an all-domain-wide guild around your data platform is favorable.
Choose the right end-to-end privacy methods: Most probably, it won’t be only one privacy method. It is crucial to ensure flexibility in what method to apply to a given streaming pipeline. The available methods include end-to-end message- or field-level cryptographic encryption. This method is useful in data migration and bridge-to-cloud scenarios and still allows for stream analytics, although at the price of higher resource utilization. Other methods leverage de-identification techniques like tokenization, perturbation, and masking.
Minimize Data Collection: Reduce the amount of potentially sensitive data stored and flowing through streaming pipelines. In the case of manufacturing or health care, companies should consider smart devices, applying edge pre-processing techniques before sending the data to, e.g., a public cloud for in-depth analysis.
Within an organization, privacy-preserving data streams allow them to connect data from different divisions. Such intelligent data aggregation may be crucial to create or train more robust and more reliable models, which can then be used for tasks like fraud detection or risk assessment in the case of financial institutions or to share and aggregate patient data in IoT-based healthcare systems.
Final thoughts on data democratization vs. data privacy
Data democratization is a concept worth taking a closer look at. It allows organizations to manage the potential of their data better and can positively influence new product development, speed-up product delivery, and increase return on investment. Consequently, streaming architectures become more widespread to maximize data utility. However, strict government and industry privacy regulations, which aim to reduce the risk of data leaks and eliminate the consequence of eventual misuse of data, could hamper this process. To remain a successful data-driven organization, businesses need to overcome challenges posed by these regulations and utilize privacy-preserving methods in their data streaming architectures. We at Mimacom would be happy to assist you during the challenging process of adopting and expanding your streaming data platforms, reducing privacy risks, and meeting compliance.
Pawel Wasowicz
Located in Bern, Switzerland, Pawel is our Head of Data Engineering. At Mimacom, he helps our customers get the most out of their data by leveraging latest trends, proven technologies and year of experience in the field.