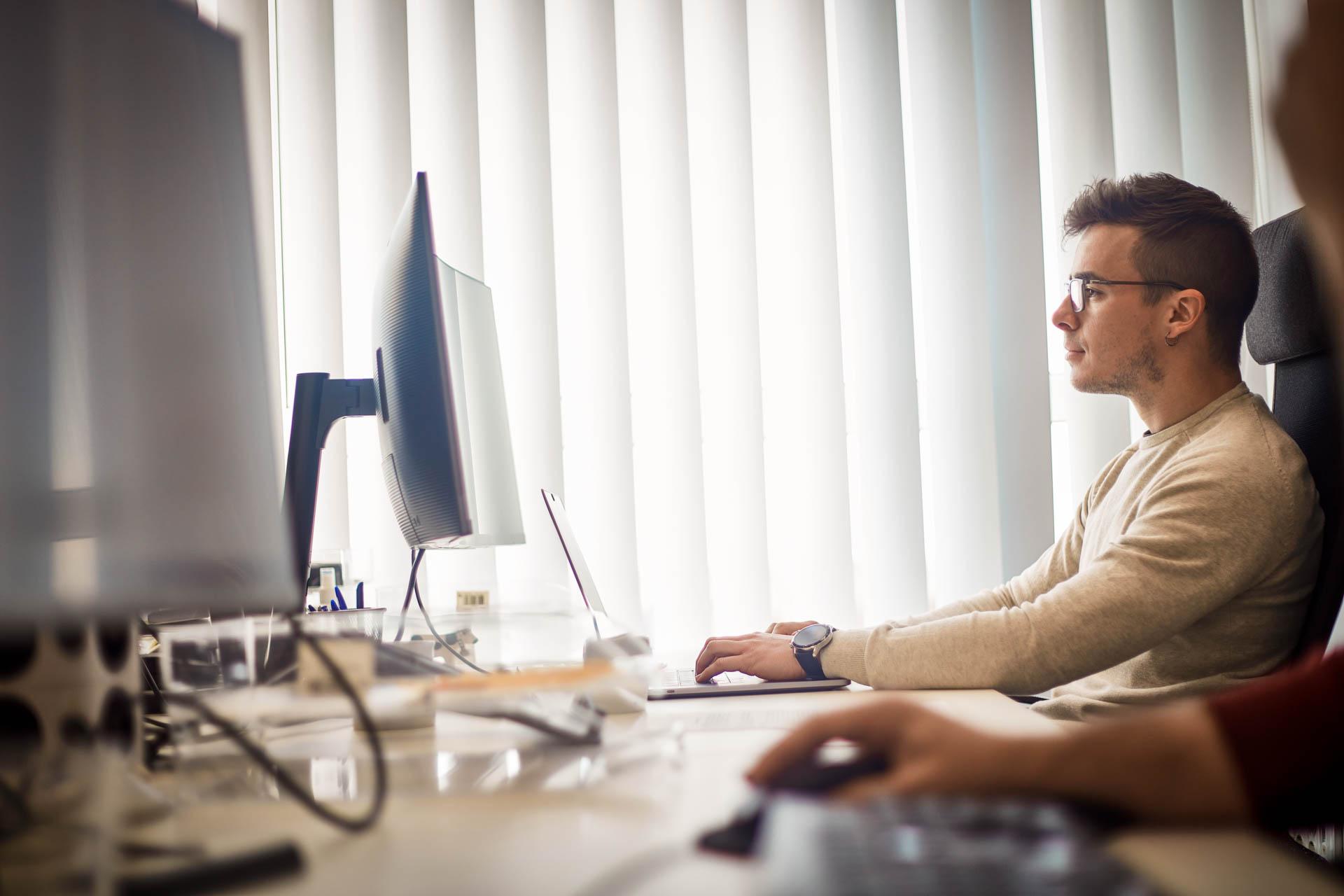
In our previous blog article about metadata management, we explained why the way of handling data has brought up a need to change the way we do it. In order to continue describing data in a meaningful way, cataloging it and visualizing it to make it understandable for business, a new, more advanced approach is needed. Learn in this article more about strategies that meet the requirements of today's data velocity, volume, and structure in metadata management. Find out how to tackle the new challenges of metadata and see how the approach of active metadata management can help you.
Common and main issues with the legacy approach
In the highly dynamic, ever-changing landscape, metadata management solutions relying on manual management fail miserably. Even if it looked manageable initially, enterprises soon realized that as the demand grew, the necessity for continuous manual interventions at every stage of this process was not maintainable.
Some providers followed the path of introducing artificial constraints to reduce or even stop possible diversity. However, it resulted in vendor lock-in and hindered further evolution. 'Gartner's "Magic Quadrant for Metadata Management Solutions" from 2020 contains an overview of these kinds of technologies.
Yet other providers followed the path of designing single-purpose solutions and exposing an API (e.g., OWL, RDF, SKOS) for higher-level integrations. This approach gave domain specialists more freedom and supported federated architectures. Consequently, decentralized responsibility was favored; however, the process remained mostly manual.
Instead of highly inefficient manual maintenance, some metadata management solutions use automatic processes leveraging agents or crawlers. These are supposed to attach themselves to data sources and push relevant information to metadata management services. Due to various data structures, agent-based designs usually suffer from surging complexity and become unmanageable. Moreover, the insufficient sophistication of these approaches makes it difficult to maintain the metadata's acuteness over time.
Description of partial solutions
There are plenty of good practices to tackle the problem. First, the metadata management layer should be developed gradually. Its evolution shall be demand-driven. The team responsible should embrace the creation of a community around it and define standards accompanied, e.g., by blueprints. Metadata management solutions shall identify and focus on the most critical and relevant data first and only after, once the pattern emerges, potentially accommodate the rest. It should define integration patterns with other systems in the organization's landscape, e.g., with the help of API via streaming. To design a sustainable solution, representatives from the whole organization shall partake in this undertaking. The metadata management layer may be a consolidated or a distributed platform or a mix of these two. It shall comply with and enforce governance rules and provide immediate access to high-quality data via a set of self-service and intelligent services.
The actual metadata store may span from ordinary key-value stores to graph databases and leverage indexing for more complex queries or even an analytics engine.
Relation to governance
Although data governance is strongly related to metadata management, it goes much further beyond the infrastructure or architectural concepts. Data governance defines and affects processes, the enterprise's organization and work culture, employees and possibly more. However, it can be said that data governance establishes policies for correctness and availability, while metadata is the way to communicate governance decisions.
The metadata management layer shall offer open and expandable access remaining fully compliant with governance policies at the same time.
Active metadata management as a solution to meet today´s metadata requirements
Metadata management is one of the central parts of modern data platforms. In 2021 Gartner recognized a change in the metadata management trends and reconsidered its original approach. After identifying issues with then-popular techniques, it highlighted a new trend: active metadata management. Recognized as a crucial concept in modern distributed data architectures, which follow principles of data mesh and/or data fabric. According to Gartner: "Active metadata management is a set of capabilities that enable continuous access and processing of metadata that support ongoing analysis over a different spectrum of maturity, use cases, and vendor solutions."
Gartner then proceeds to identify essential parts of it:
ML instead of profiling
A process of defining metadata associated with data sets was hierarchical and leveraged statistical algorithms operating on attribute-level, cross-column, or even cross-dataset. This was commonly described as data profiling. This approach does not scale well with the number of datasets and is not flexible enough. According to Gartner, a technique used to analyze and gain a better understanding of raw data shall leverage machine learning algorithms and it should be used as the first step in determining what insights data can yield.
Content analysis
It is a technique used to make replicable and valid inferences by interpreting and coding textual material. Applied systematically, content analysis tools may provide actual quantitative data, effectively representing any content in a measurable way. This then lays the ground for actionable insights.
User/ Use case clustering
By finding patterns in data usage, one can proactively detect and enable potential new use cases to increase the performance of existing ones. This capability includes grouping users with similar viewing patterns to recommend similar content supporting anomaly detection techniques.
Resource allocation metrics
Having extensive metrics related to resource measurements is crucial for the dynamic and autonomous allocation of software and hardware resources.
Alerts and recommendations
The operationalization of analytics comes in the form of alerts and notifications. Insight becomes immediately available.
New asset inference
The process of finding non-explicit relationships and structures in data. Continuous comparison with passive metadata helps to apply quality rules and provides recommendations for users to support metadata discovery.
Orchestrate recommendations and responses
Interoperability with data management platforms is crucial for providing more complete answers. Integrating design and runtime metadata across data platforms make it possible to infer new information.
Active metadata management is a chance for high-quality data access and governance compliance
Previously proven approaches are no longer suitable to handle today's metadata management. In this article, we explained different approaches to managing the new challenges handling data has brought to metadata management. The metadata management layer may be a consolidated or a distributed platform or a mix of these two. It enables compliance with governance rules. Moreover it shall provide immediate access to high-quality data via a set of self-service and intelligent services. Gartner has highlighted active metadata management as a new trend and a crucial concept in modern distributed data architectures and with this post we highlighted the essential parts of it. In our next article about metadata management, we will explain the principles of metadata management in data fabric architectures.
Pawel Wasowicz
Located in Bern, Switzerland, Pawel is our Lead Data Engineering within Digital Foundation. At Mimacom, he helps our customers get the most out of their data by leveraging latest trends, proven technologies and year of experience in the field.