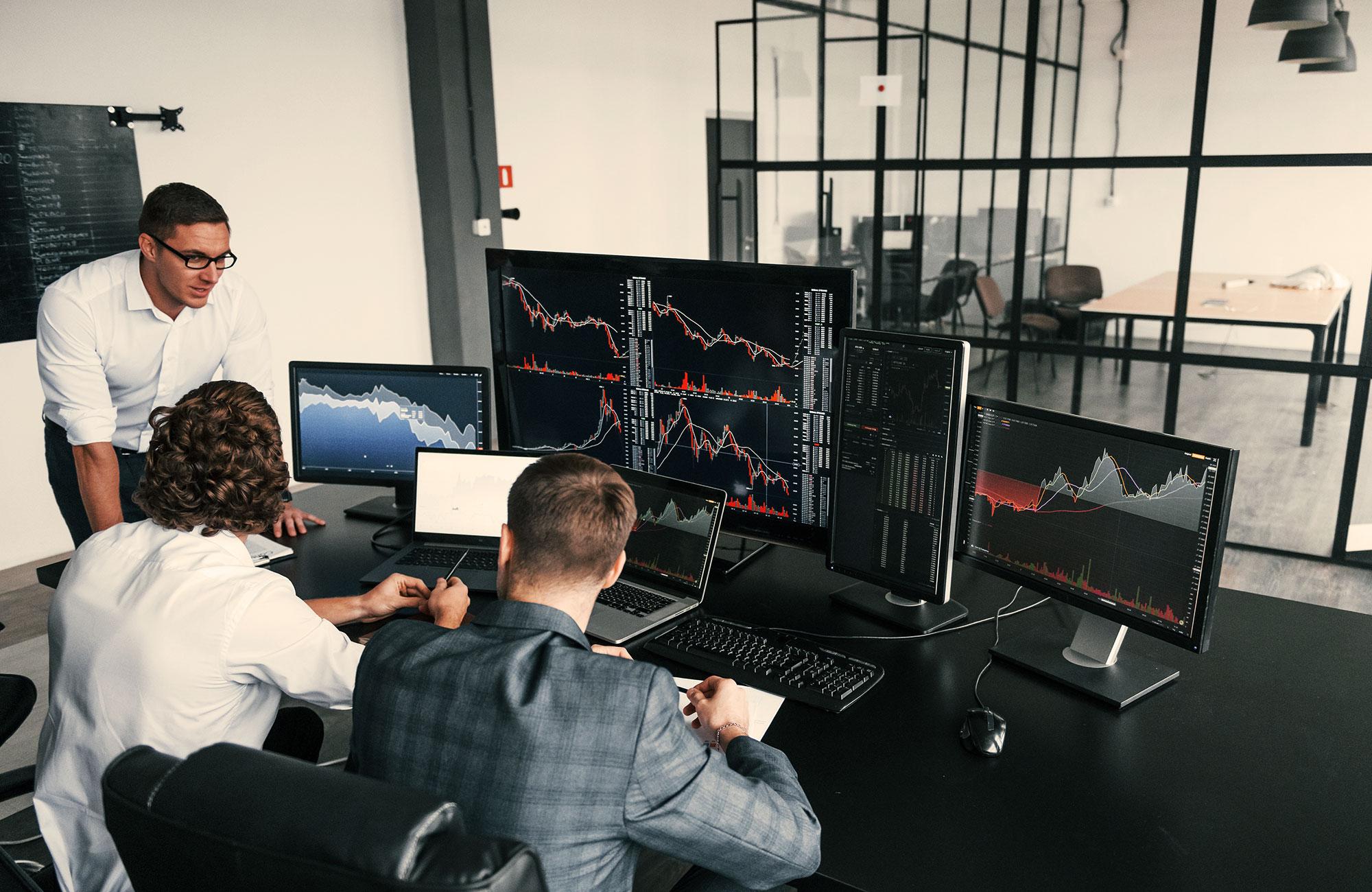
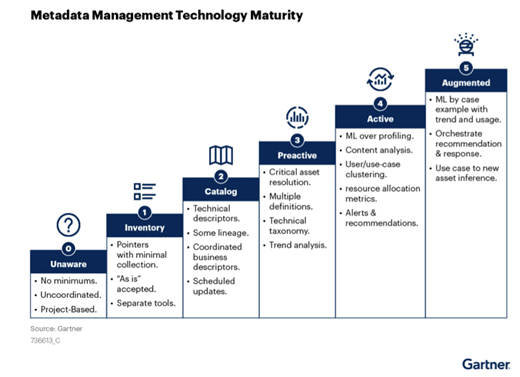
Metadata management in data fabric
In the second blog article of the metadata blog series, we explained why previously proven approaches are no longer suitable to handle today's metadata management. As Gartner has highlighted active metadata management as a new trend and a crucial concept in modern distributed data architectures, we highlighted the essential parts of it. In this third and last article about metadata management, we will explain the principles of metadata management in data fabric architectures.
Metadata Technology Maturity Model
According to Gartner, the evolution to active metadata management architectures follows a few stages.
It starts with ad-hoc and uncoordinated metadata management, where no standards exist yet and projects are fully independent in their approach. Then it evolves by defining organization-wide standards practices, leveraging dedicated tools enabling first insights focused on dataflows and lineage. It finally reaches the level where machine learning techniques may be used to provide desired active metadata management capabilities described above.
Every step in this process needs to be carefully planned and performed with a clear vision in mind. It is good to rely on the experience and know-how of companies like Mimacom during this transition.
Based on the presented market research, augmented data management capabilities are of central interest. This may be seen as the main driving force behind the paradigm shift in metadata management platforms. Gartner does not deem passive metadata management systems doomed but predicts autonomous systems like those underlying active metadata management to gain considerable momentum. The fundamental block of active metadata management is going to be AI.
Metadata management is a part of Data Fabric architecture
Modern architectures of data platforms face many challenges. As mentioned at the beginning, data lake architecture, while it addresses the need for non-homogeneous datasets, does not easily accommodate neither the requirement of multi- or hybrid-cloud nor federated domains solutions.
Data platform architecture like data mesh or data fabric embrace Domain-Driven Design concepts hence favoring distributed, independent data domains, address the needs of integrating multiple technologies and tools.
Data fabric and mesh concepts address all the problems that arise in modern, complex, non-homogenous data platforms but choose slightly different approaches. Both designs aim at delivering holistic data insights. However, while data mesh focuses on distributed, domain-centered data stewardship, data fabric places seamless integration and data sharing in the middle.
Data mesh architecture strongly emphasizes DDD and is API-driven; hence it favors project independence and relies on higher-level integration. Data governance compliancy in this approach is enforced separately and the final data governance layer is emergent. Data fabric concept, while still embracing distributed and domain-driven architectures may be described as a more low-code solution driven by AI augmentation.
The differences are more visible when it comes to metadata management. Data governance, which metadata management is a part of, has a bottom-up structure in data mesh. It relies on domains’ responsibility, remains federated and gets integrated leveraging central arrangements. In data fabric architecture, as it focuses on data management and security from the beginning, governance is supposed to be a built-in feature.
As usual, it does not mean organizations have to choose one or the other approach. Both concepts: fully domain-based data mesh with emerging governance and data fabric with its augmented active metadata management solution can coexist. It depends on the specific needs of any enterprise to find the proper balance between both architectures.
Conclusion
In this blog series, I focused on metadata management in data platforms. I showed how the original passive metadata management does not fit the needs of modern data platform architectures and why it must be enhanced or even replaced with autonomous processes of active data management approaches. I then proceeded to make a short comparison of approaches to data governance, in which metadata management is crucial in two leading data architectures: data mesh and data fabric. Both offer promising remedies for issues related to the ever-growing complexity of data platforms.
We at Mimacom, would be more than happy to help you find the right path for a data platform and its evolution in your organization.
Glossary Metadata Management
Data lineage: It is a way to understand how data flows through the whole platform. It is supposed to provide multi-dimensional insights: what systems and when participating, how it is transformed and processed within a single system and finally, how it relates to business processes.
Data provenance: A crucial part of lineage as it deals with the origin of data, i.e., data capture layer and data sources.
Data governance: It is a set of activities that involve description, design, implementation and enforcement of control over data and data management processes. It relates data with data ownership or stewardship, is closely related to data security and relies strongly on metadata.
Data mesh: An architectural approach to data platforms. Naturally, the next step in the evolution of the data lake. Following Domain-Driven Design concepts embraces distributed architectures, flexibility and reasonable project independence while maintaining accessibility and availability of data at the same time.
Data fabric: Is an architectural approach aiming at providing seamless access to data in a way that is compliant with required regulations leveraging autonomous techniques.
Data democratization: Is an approach to bring data closer to all interested. Providing them with tools and services to find and use data more efficiently. This trend may leverage self-service portals for data discoverability and provisioning, marketplaces making use of all kinds of metadata and intelligent services supporting various decision-making processes.
Schema-on-write vs schema-on-read: Schema-on-write is a procedure of defining data structure before writing to DB. It is an original approach popular with RDBs. Schema-on-read, on the other side, is a more agile approach where the schema is defined while consuming data. Schema-on-read speeds up the process of onboarding new data sources.
Pawel Wasowicz
Located in Bern, Switzerland, Pawel is our Lead Data Engineering within Digital Foundation. At Mimacom, he helps our customers get the most out of their data by leveraging latest trends, proven technologies and year of experience in the field.